All sports and games are ultimately based on a combination of math and logic. Soccer, baseball, tennis, chess and (in card games,) other players will all analyze their opponents’ statistically weak points in order to develop tactics and plays to target them. When it comes to recreational gambling, math can be a helpful tool in developing basic and advanced strategies for online casino games such as blackjack and roulette. At the poker table, working knowledge of the various types of poker odds has multiple advantages.
The relevant branch of math, in this instance, is called probability theory. Beyond knowing how to play poker, professional online poker gamers know how to calculate the probability in poker of various hands winning in various situations. This post details the two types of probability theory — classical or frequentist and Bayesian — and outlines how Bayesian inference specifically can enhance your chances of winning when you play online poker.
What Is Probability Theory?
Probability theory can be applied in a vast range of domains, ranging from simple scenarios such as rolling dice or flipping coins to complex situations such as political elections, epidemics, financial speculation, economics and more. Underpinning it all is the idea that outcomes contain a random factor, an element of chance beyond any agent’s ability to determine or control. Poker players will readily appreciate this concept. Unlike a game such as chess, where players theoretically have complete information (even if only the most skilled players know how to obtain and use it,) poker is a game of strategic decision-making under circumstances of incomplete information where chance plays a significant role.
Classical Probability vs Bayesian Inference
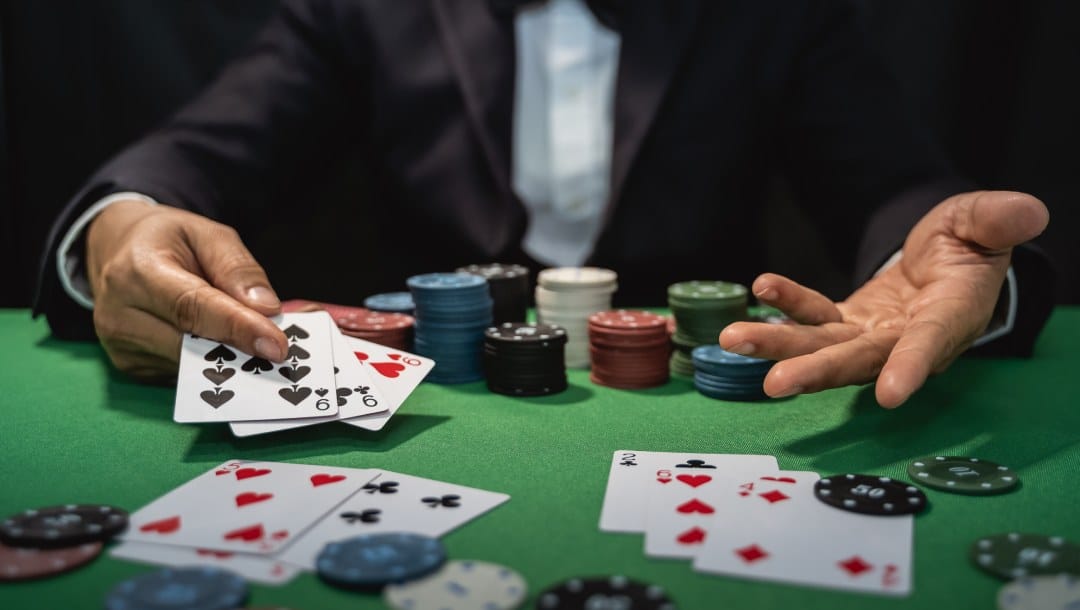
Statistical inference is when you analyze data and draw conclusions based on random variation. The type of statistical inference that most people are familiar with is known as classical or frequentist statistics. This assumes that probabilities are based on how frequently particular random events occur in a long run of repeated trials. Dice rolls are a good example. If you roll a six-sided die repeatedly over a long time, each number tends to come up one time in six (assuming, of course, that the die isn’t weighted to favor a specific outcome.)
The philosophy behind frequentist statistics is that it’s possible to obtain certainty in the abstract by providing estimates. In this case, it’s an abstract certainty that, on average, a die will roll a six one time in six. The trouble is that you can’t really rely on this information to predict the outcome of a specific roll at a craps table, for example.
Bayesian statistics has a different philosophy. Instead of trying to eliminate uncertainty, Bayesian inference tries to refine uncertainty by updating an individual’s pre-existing beliefs about random events as new data or evidence concerning them comes to light. Under this theory, probability is a measure of how confident an individual can be that a specific event — say, a poker opponent bluffing on the river — will occur.Â
Coin Flips
As an example, compare frequentist and Bayesian statistics. Say, you’re flipping a coin and want to know the probability of the coin coming up heads. What you don’t know is that you’re actually flipping an unfair coin — it’s weighted to come up heads the majority of the time.
Under frequentist statistics, you would carry out repeated flips of the coin and measure the long-run frequency of seeing a head. Eventually, you determine that this specific coin is statistically certain to come up heads a certain percentage of the time. The important thing is that your belief in the fairness of coins in general doesn’t count.
Under Bayesian statistics, your beliefs do count. Before you flip the coin, you may believe that — based on personal experience and general knowledge — this coin is fair. But you flip it a few times and it keeps coming up heads, so you think that maybe it isn’t a fair coin. To test the theory, you flip it 500 times and the outcome is heads 400 times. Now you’re convinced that this coin is very likely to be unfair. In other words, you’ve rationally updated your personal beliefs about the situation based on new information — a rational choice to update a belief that ChatGPT can’t make.Â
Applying Bayesian Thinking to Online Poker Games
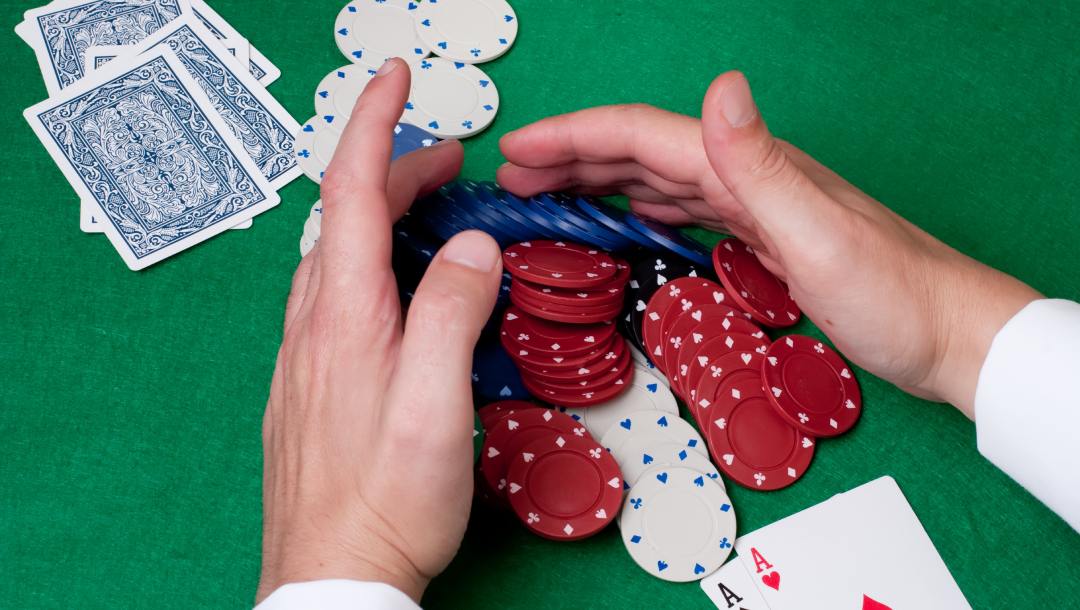
Probability is enormously important in online poker, helping players decide whether to bluff, call, raise, fold or bet. In practice, both types of probability theory have their place in poker, but Bayesian theory has broader applications.
Say, you’re playing Texas Hold’em and you’re holding four of a kind after the flop. According to classic probability theory you can be certain that the probability of an opponent beating your hand with a straight flush or royal flush is only 0.000015, so you can bet with confidence — odds that vary slightly in Omaha, although the principle remains the same.Â
The trouble is that in poker, things are rarely as clear-cut as that because it is also necessary to take all your opponents’ previous actions into account and update your take on the probability of their actions accordingly.
Say, the texture of the board you’re currently playing is wet and there’s a possible flush. You think your opponent may have the flush or they could be bluffing. You put them on a range where there are 100 possible hand combinations they could get to the river with. Of these combinations, 20 are flushes, 30 are combinations such as top pair or two pair that they wouldn’t bet or bluff with, while 50 are busted hands they could use to bluff. They then bet $500 into an $800 pot. Do you call?
The pot odds dictate that you need to win 28 percent of the time for a call to be worthwhile. Assuming your opponent bets all 20 flush combinations, they have to bluff with at least another eight combinations out of this total pool of 50 possible bluffing hands. So how often does your opponent actually pull the trigger on a bluff? If you’ve observed them and you know it’s, say, at least 20 percent of the time, then there’s a conditional probability that they’ll bluff with at least eight combinations. This is enough for you to call, because you’ll win eight times out of 28.
Play Poker Online at BetMGMÂ
Notice that this blog post doesn’t include any mathematical formulas? That’s because actual Bayesian probability calculations require advanced training beyond the scope of this blog. But you can apply Bayesian thinking to your poker game immediately. Simply register at BetMGM and get started with cash games and online poker tournaments with stakes and buy-ins appropriate to your budget. Observe your opponents, apply the principle of conditional probability and watch your game improve.